Competitive Innovation is Improving the Economics of GenAI
At this stage of the development of Generative AI, there’s much we can see clearly (at least we think we can), but there’s even more likely to surprise us. As that great 20th-century philosopher and hall-of-fame catcher, Yogi Berra, famously said, “It’s tough to make predictions, especially about the future.” The breadth and speed of innovation today in all aspects of GenAI rivals that of the internet in the 1990s, and for the same reason.
Most of the necessary foundational elements were already in place when ChatGPT raised public awareness of artificial intelligence almost overnight. This event galvanized a broad, competitive effort to exploit the potential of the technology to its fullest. ISG Research asserts that, by 2027, almost all providers of software designed for finance organizations will incorporate some AI capabilities to reduce workloads and improve performance. Because so much is happening out of sight of one individual’s area of immediate expertise, and because so many improvements in the technology are individually small but in aggregate constitute important advances, there is a constant stream of unexpected reverberations from events that change the outlook for the practical uses of GenAI in business.
As has been the case in every major technology shift, economics is the invisible hand controlling how GenAI is applied. The cost of GenAI-driven capabilities will determine when, where and to what extent enterprises will use it, either through proprietary initiatives or in GenAI-enabled applications. Recent announcements suggest that a declining cost curve might be steeper in the near term than expected by the skeptical. This includes open-source models' ability to compete closely or on par with proprietary models, improved techniques for using synthetic data in training models and model distillation. All of these may make a broader set of business computing use cases cost-effective sooner.
First, some background on the cost of AI and its impact on demand and adoption.
A year ago, I raised the question of how the cost of generative AI would affect demand, supply and where those costs would be borne across the full value chain. At that point, few were raising the question because we’ve grown accustomed to these sorts of costs being more than made up for by gains in productivity or other sources of value or socialized, like the cost of email. Over the past year, because of the eye-watering sums spent on the hardware needed to evolve and support GenAI, questions have been raised as to whether all its potential applications can be realized over the next three to five years because many may fail a cost/benefit test.
ISG Research recently quantified attitudes around spend in our Market Lens AI Study, which asked participants how much more they would be willing to pay for AI capabilities. The research provides insight into how enterprises currently expect to gain value from AI and, therefore, the propensity to pay for AI capability in applications.
The research finds the greatest inclination to spend is in sales performance management, which I interpret to mean that the participants see this area as having the highest potential to generate profit through gains in sales productivity and, therefore, increase revenue. The next five include supply chain management (to cut costs), treasury and risk management (for more accurate cash flow forecasts, to reduce risk of fraud and credit losses as well as cut the cost of regulatory compliance), IT service management (to cut costs), analytics and business intelligence (to gain productivity) as well as procurement (cut costs). In other words, enterprises are willing to pay for productivity gains that clearly generate revenue and easily achieve cost savings or reduce risk. Those offerings that promote productivity without a direct connection to increased revenue or cost savings, or where the cost savings are perceived to be limited or difficult to achieve, will not make the cut until investments become economically attractive.
Recent developments suggest that the race for market share is driving innovations that can bring about a greater range of practical and affordable GenAI advances in business computing sooner.
The release of Meta’s Llama 3.1 brings this open-source large language model roughly on par with proprietary models. The open-source computing model has been very successful because it’s cost-efficient and fosters rapid innovation through collaboration. Over time, the risks associated with its use (such as security and intellectual property issues) have proven manageable.
A major advantage of this latest release is that it permits users to train and fine-tune models of varying size on their data without exposing it. Moreover, constantly training models on an enterprise’s real data prevents model collapse, a degenerative process caused by indiscriminately learning from data produced by other models. In essence, accurate modeling is forever in need of a reality check.
The ability to economically and efficiently train and fine-tune models of various sizes for a specific use case is essential for business applications. Size matters because, for business software, some tasks in a process need a large model, but others can be more cost-effectively supported with a narrow language model or through an orchestration of narrow models. For example, a set of narrow models might be the best approach to automating the “reading” of a digital invoice attached to an email, summarizing or extracting elements of the message in the email it is attached to, and performing the transaction recording and accounting. This automation not only boosts productivity at the front end of the process but also substantially reduces data entry errors, provides important context that is otherwise lost and cuts down on the need for internal audit and other quality control efforts.
Competition is having an impact on pricing. Following Llama 3.1’s release, OpenAI has fine-tuned GPT-4o Mini free. Open source also creates pressure to develop efficiencies that drive down provider costs but also structure pricing on a value chain that better reflects market demand. Rather than being a one-price bundle, services and products are disaggregated. Some basic elements become free of charge, while others are priced to reflect the value provided to the customer. This market-driven impact on pricing reduces inefficiencies and accelerates adoption and consumption.
The ability to apply model distillation has, up until now, been prohibited by the terms of service of all providers. Distillation is a technique where “student” models are trained to mimic a larger and more complex “teacher” model. Student models are smaller and simpler and, therefore, less expensive to operate because they consume fewer compute resources. They make pre-training faster and less expensive than using a larger model to achieve sufficiently satisfactory results. Llama 3.1 permits distillation with limited restrictions, which will probably force others to follow suit.
Despite the longer-term promise of more affordable GenAI applications, enterprises seem hesitant to make major commitments. This might be because the rapid rate of change in the technology is creating too much uncertainty and, therefore, perceived risk. Paradoxically, GenAI’s stunning early success across the board has tended to freeze the market to a noticeable degree. However, the impact of this reluctance appears mainly for internally developed projects because software providers have been moving as rapidly as possible to infuse AI features and capabilities into software.
I strongly recommend that senior leadership teams have a clear understanding of the benefits of AI and GenAI, how to achieve them and what they’re worth. We are on the cusp of a generational change in the tools available to enable enterprises to do more and improve performance. Despite claims to the contrary, business challenges never change. It’s the tools available to conduct business that are always evolving, providing those clever enough to use them with a competitive advantage that challenges others. CFOs, in particular, must be prepared to incorporate AI across their department, yet most are ill-prepared to adopt new tools and their methods. Our Office of Finance Benchmark Research finds that 49% of departments are technology laggards while just 12% are innovative. This matters because the research also shows a correlation between technological competence and how well the department performs core processes. CFOs need to adopt a fast-follower approach to using AI and GenAI—adopting technology as soon as it is proven to enable their entire organization to stay ahead of the pack.
Regards,
Robert Kugel
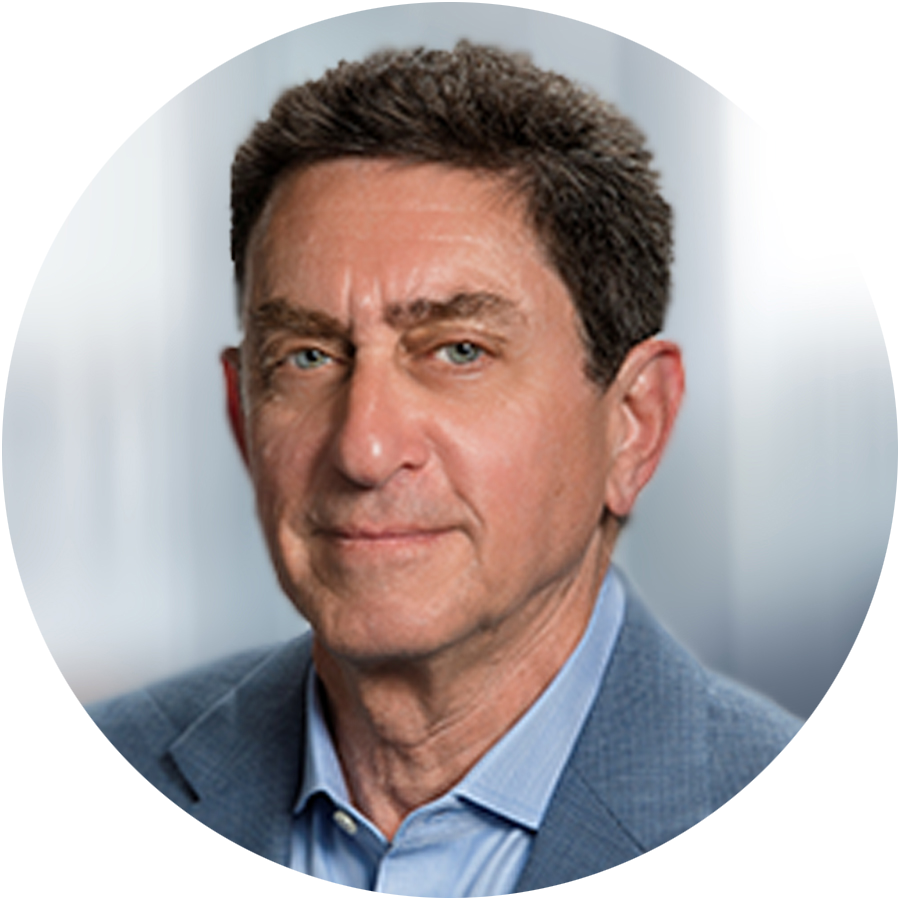
Robert Kugel
Executive Director, Business Research
Robert Kugel leads business software research for ISG Software Research. His team covers technology and applications spanning front- and back-office enterprise functions, and he runs the Office of Finance area of expertise. Rob is a CFA charter holder and a published author and thought leader on integrated business planning (IBP).